Evaluating Financial Performance Metrics: Enhancing Traditional Models with Behavioral Insights
As a Research Assistant under Dr. Jens Klinker at the Technical University of Munich, I worked on advancing financial performance metrics, focusing on integrating behavioral finance principles and addressing shortcomings in conventional evaluation models like the Sharpe Ratio. Our work aimed to propose a novel metric that balances performance evaluation with nuanced considerations of volatility and market dynamics.
Research Motivation and Objectives
Traditional financial performance metrics such as the Sharpe Ratio, Treynor Ratio, and Jensen's Alpha have limitations when contextualizing performance relative to broader behavioral and market conditions. For instance:
- Sharpe Ratio: Focuses solely on excess return per unit of risk but overlooks comparative performance across similar securities.
- Treynor Ratio: Highlights market correlation but is less intuitive when ranking individual securities within a cluster.
- Jensen's Alpha: Attempts to mathematically encapsulate market performance but can overly prioritize returns while downplaying volatility.
To address these gaps, our objective was to refine these metrics by introducing a cluster-based ranking system, coined "PRISM" (Performance Risk-Integrated Stock Metric), that evaluates securities relative to peers with similar volatility profiles.
Methodological Approach
The PRISM framework builds upon existing models by incorporating clustering and percentile-based rankings:
- Cluster Formation: Securities are grouped based on volatility within a defined range (e.g., ±2 standard deviations). This grouping mitigates skewed comparisons between highly volatile and stable stocks.
- Performance Adjustment: The difference between a stock’s performance and its cluster median is calculated, ensuring peer-relative evaluation.
- Volatility Integration: The performance difference is normalized by dividing it by the stock’s volatility, emphasizing risk-adjusted returns.
- Percentile Ranking: Stocks are ranked based on their adjusted performance, facilitating intuitive comparisons across the market.
Addressing Methodological Challenges
A critical issue arose with clusters of size one, where no meaningful comparison could be made. To mitigate this, we refined the clustering algorithm to enforce minimum cluster sizes, dynamically adjusting boundaries until a sufficient number of securities were included.
Integration of Elo Ratings
An interesting extension of this methodology is the integration of Elo Ratings, commonly used in ranking systems like chess. By applying an iterative Elo-like approach to update cluster boundaries dynamically based on stock performance, we could achieve a more robust, real-time adaptation to market shifts.
Key Results and Insights
Our research demonstrated that PRISM offers a more balanced perspective by:
- Reducing bias from extreme market outliers.
- Enabling intuitive percentile-based rankings for investors.
- Highlighting securities that outperform peers on a risk-adjusted basis.
- Presented results to Prof. Dr. Tim Kroenke’s Lab at the Université de Neuchâte.
Additionally, comparative analysis revealed strong correlations between PRISM and traditional metrics like Sharpe and Treynor. However, PRISM provided additional granularity in distinguishing securities within similar risk profiles.
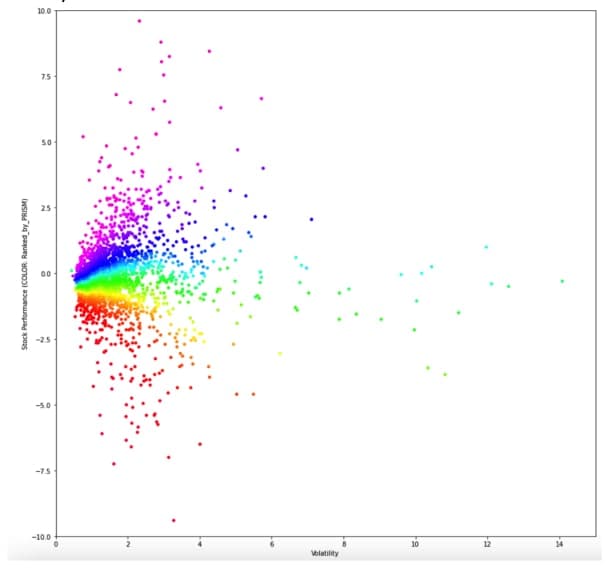
Figure 1: PRISM Performance Visualization This scatter plot demonstrates the distribution of stock performance (adjusted by PRISM) against volatility, with colors representing percentile rankings. The visualization highlights PRISM’s ability to differentiate stocks based on risk-adjusted returns within similar volatility clusters.
My Contribution as a Research Assistant
Under Dr. Klinker's guidance, I contributed to:
- Designing the PRISM algorithm, including the clustering and ranking mechanisms.
- Identifying and addressing logical inconsistencies, such as cluster size constraints and their impact on performance evaluations.
- Visualizing the results to effectively communicate findings to academic and industry stakeholders.
Reflections and Future Work
This project underscored the importance of adapting traditional financial models to better reflect nuanced market realities. Future work could explore integrating machine learning for dynamic cluster formation and applying PRISM in portfolio optimization strategies.
Through this research, I gained invaluable experience in quantitative finance, data analysis, and algorithm development, furthering my commitment to developing innovative solutions at the intersection of finance and technology.
While this project provided me with valuable insight into using computer simulation to tackle theoretical questions, I ultimately found that its potential was constrained by certain limitations. The theoretical framework, while ambitious, lacked the depth needed to fully bridge the gap between simulations and complex real-world economic systems. Additionally, the reliance on simplified models made it challenging to achieve results that could be broadly applied. While the project offered intriguing conceptual findings, its focus on abstract scenarios limited its practical impact. These challenges, combined with my interest in pursuing research with more direct applications and my limited background in economics, ultimately led me to shift my focus to a different area.